RESEARCH
My scientific research tackles understanding the immune variation of infectious/inflammatory diseases through host genetic, cellular and statistical analyses at the population level.
Host genetic factors
First, we identified HIV+ progressors (slow, rapid, intermediate) through a retrospective epidemiological analysis. The figure below on the right shows the main clinical data used for the selection of individuals. You can find more details about the design of the characterization of progressors in our publication (de Medeiros et al. PlosOne, 2016).
Then, through different studies in our group, we selected potential SNPs on different genes regulating the different layers of HIV pathogenesis. Since we intended to understand each SNP's size effect contribution and how they interact. We used the Multifactor Dimensionality Reduction (MDR) algorithm, a non-parametric statistical analysis, to assess the gene-gene interaction shaping the phenotypes. We found a significant interaction between SNPs of CXCL10 and CCL22 in the susceptibility to HIV infection and between IL-10 and TLR9 genes. Also, we contribute to our understanding of considering the genetic background of individuals in an admixed population. You can find our publications regarding this topic here.
Immune factors
We characterized the inflammatory profile of HIV+ individuals in the different stages of the disease. We suggested IL-10 and IL-6 as important biomarkers of the pre-AIDS phase in slow progressors (SPs) and rapid progressors, RPs (de Medeiros et al. PlosOne, 2016).
Then, since we followed up with individuals before and after the initiation of antiretrovirals, we identified significantly high levels of pro-inflammatory proteins in individuals under treatment, such as CXCL10, CCL20 and IL-8. The CXCL10 chemokine is crucial early in the infection, but its persistence at higher levels can potentially be harmful to the organism, as observed by many other studies in other viral infectious diseases. The IL-8 is an important cytokine for the chemotaxis of immune cells and angiogenesis and participates in the activation and differentiation of hematopoietic stem/progenitor cells. This cytokine is also a hallmark of chronic inflammation, associated with different outcomes and non-communicable diseases like cancer or cardiovascular diseases. We suggested these cytokines as potential biomarkers of chronic inflammation in HIV+ individuals (Valverde-Villegas et al. Infect Genet Evol, 2018; Ellwanger et al. Microbes Infect, 2020).
Overall, recovering the immune balance after infection depends on various factors, including the immune state of individuals early in the infection host genetic and viral factors. In the context of the timing treatment, we observed that individuals who initiated the treatment early in the infection recovered better the immune balance when analyzing some pro-inflammatory cytokines, T cell subsets and cells of immune activation) compared to whom initiated the treatment late in the infection.
My research also aimed to identify and characterize hematopoietic stem and progenitor cells (HSPCs) in the breastmilk compartment of HIV+ women from Zambia, Africa. Why? Because these cells from peripheral blood are considered reservoirs for HIV latency. After diving deeper into the stem cell world, I implemented my protocols to characterize these cells using the two primary stem cell markers, CD34 and CD133 along with CD45. There is a high heterogeneity of these cells, and the switch of stemness marker expressions relies on the differentiation of these cells, tissue-specific and its microenvironment. It was fascinating to be aware of this complexity and how it may impact their permissiveness or resistance to HIV and other viruses. I identified different cell subsets by combining these markers through a boolean gate strategy, and importantly we reported different frequencies when compared with its counterpart blood and when depending on the lactation stage (colostrum and transitional milk). Still, our publication only reports the most quantifiable population since these cells are rare, especially progenitor cells (Valverde-Villegas, et al. J Hum Lact, 2020). I also identified significantly higher frequencies of CD133+ cell subsets in blood and milk compartments of HIV+ women vs. HIV- women. By contrast, significantly lower frequencies of CD34+ cell subsets were observed in only the blood of HIV+ women vs. HIV- women (Valverde-Villegas et al. Front Cell Infect Microbiol, 2020), as observed in other studies. Overall, there are different stem cell phenotypes in the breastmilk compartment, and the CD133+ cell subsets are increased while CD34+ cell subsets are decreased upon HIV infection. More studies are needed to assess the mechanistic insights behind the stemness and different cellular distributions against viral infections like HIV.
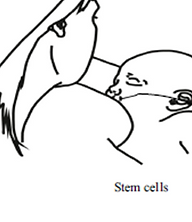

(Stages of breastmilk, figure from milkbarbreastpumps.com.au)
Breastmilk
We also found positive correlations with pro-inflammatory cytokines, such as CXCL10 and CXCL12, and the frequency of CD133+ cell subsets in HIV+ samples with detectable and undetectable viral load (check here). Cytokines are essential in the differentiation of stem cells, development of mammary glands and milk production, but viral infections might potentially impact the unbalance of this inflammatory profile, so how is this microenvironment in health and disease "smouldering" on the cell phenotypes? How is it turning out to be more permissive or resistant to pathogens? Which cell phenotype from a particular stage of lactation is less or more susceptible? Are they reservoirs for HIV latency? We wanted to answer some of these questions but were challenged to gain deeper insights into the molecular mechanisms since we needed to collect new and more samples. Likewise, we opened new avenues in the field of stem/progenitor cells and infectious diseases. More studies are necessary to understand the molecular mechanisms of these cells in health and disease. Nowadays, we have cutting-edge technologies to assess single cells and their microenvironment.
Statistical genetic analyses
Since we implemented new protocols for genotyping potential SNPs associated with susceptibility to HIV and disease progression, we applied new statistical approaches to analyze the interaction between them (epistasis?) and to assess how they are distributed among the phenotypes and how we can infer the size effect of each SNP. Besides using multivariate regression, we applied a non-parametric algorithm, the Multifactor Dimensionality Reduction (MDR). We found this approach accurate to analyze a small group of individuals very well characterized. Of course, it is always much better to increase our sample size. Examining interactions is a promising research area that can reveal new biomarkers predicting patient outcomes. The figure below shows how MDR works to estimate these interactions. You can find more about MDR in the work of Neil et al. Front. Genet. (2015) and our work (Valverde-Villegas et al. AIDS, 2017).
MDR
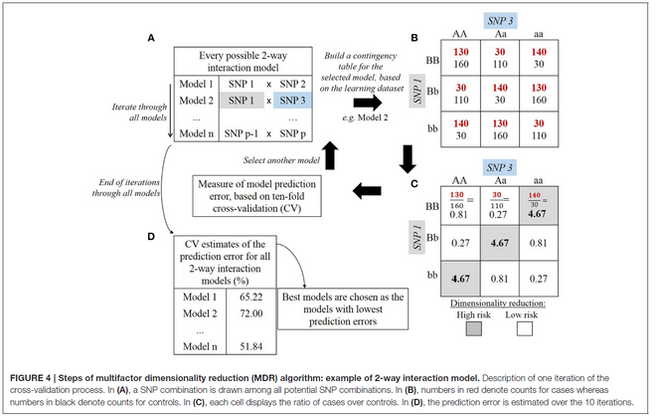
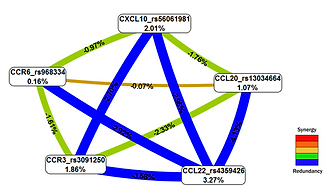
SNPs interaction in the susceptibility to HIV infection.
(Valverde-Villegas, et al. AIDS, 2017)
(Neil et al. Front. Genet, 2015)
I was recently trained to dissect GWAS data using the Mendelian Randomization approach at Cambridge University. This statistical analysis is designed to allow us to make inferences of causality. This method uses genetic variants as instruments to make inferences about the causal effect of an exposure on an outcome. This exposure can be a biomarker, an anthropometric measure, a dietary or lifestyle factor, or any other risk factor that may affect the outcome. In two-sample Mendelian randomization, the variant–exposure associations are estimated in one dataset, and the variant–outcome associations are estimated in a second dataset. The Two-sample investigations often occur when genetic associations with the exposure are estimated in a cross-sectional sample of healthy individuals to reflect genetic associations with usual levels of the exposure in the population, and genetic associations with a binary disease outcome are estimated in a case-control study (Burgess et al. Eur J Epidemiol, 2015). We can now explore published OMIC datasets and GWAS data to investigate causality through MR.
Future directions:
The complement of both genetic statistical approaches offers unique opportunities in the human genetics field to look for all potential genetic interactions in such datasets and then to make causal inferences of these interactions in epidemiological studies. I am excited by applying these approaches in my future projects.